AI Ethics in Business Intelligence: Responsible Data Collection
Explore ethical AI in business intelligence, covering data privacy, transparency, responsible scraping, and regulatory compliance for responsible automation.
Posted by

Related reading
How AI is Revolutionizing Subsidiary Compliance Monitoring
Explore how AI automates subsidiary compliance monitoring, from tracking certifications to regulatory updates, and improves risk management.
The Future of Automated Business Intelligence in 2025
Discover trends in BI automation for 2025, including multi-modal AI, real-time monitoring, and natural language advancements shaping business research.
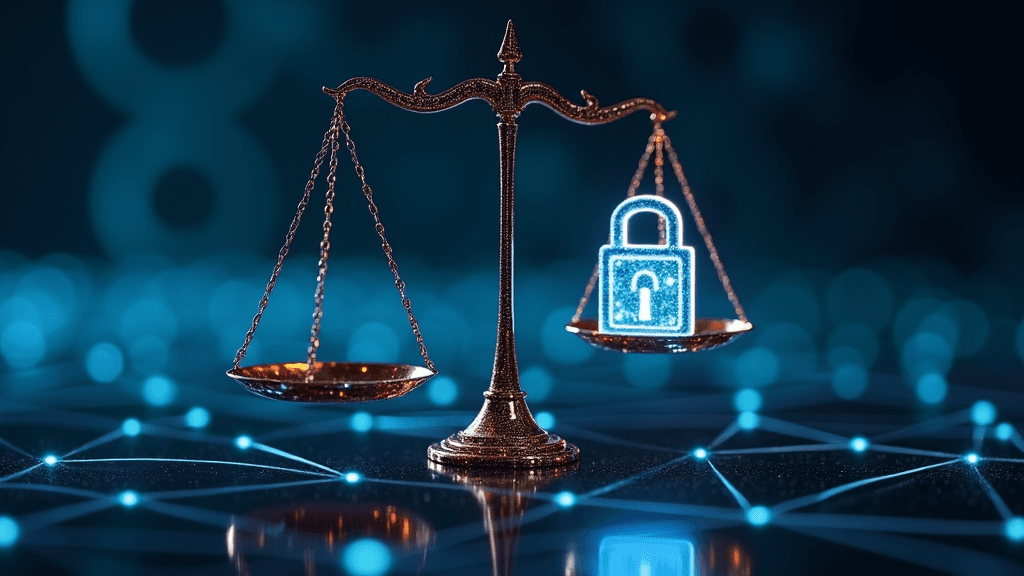
Introduction
The rise of AI in business intelligence (BI) has transformed how companies gather and analyze data. However, with great power comes the responsibility to ensure ethical practices. From respecting data privacy laws to maintaining transparency in AI decision-making, organizations must navigate a complex ethical landscape. This article examines best practices and regulatory considerations for deploying AI responsibly in BI.
The Importance of Ethical Data Collection
Ethical data collection is essential to maintain trust and comply with legal standards. Key principles include:
- Respecting privacy: Adhering to data protection regulations like GDPR and CCPA.
- Transparency: Clearly documenting how data is collected, processed, and used.
- Fair use: Avoiding exploitation of website resources or violating terms of service.
Responsible Scraping Practices
Web scraping, a cornerstone of BI, requires careful handling to ensure ethical practices. Guidelines for responsible scraping include:
- Honoring robots.txt: Respecting website guidelines on automated access.
- Limiting server load: Using rate limits to minimize the impact on website performance.
- Attribution: Citing sources when using extracted data to ensure transparency and credibility.
For example, AskMyBiz incorporates compliance checks into its systems to ensure that scraped data aligns with ethical standards.
Balancing Automation and Respect
Automation can create tension between efficiency and respect for digital resources. Best practices to maintain balance include:
- Selective scraping: Focusing only on relevant data to reduce unnecessary bandwidth usage.
- Engagement with site owners: Building partnerships or seeking permissions when appropriate.
- Monitoring impact: Continuously evaluating the system’s footprint to ensure minimal disruption.
Transparency in AI Decision-Making
Transparency builds trust and accountability in AI systems. Steps to enhance transparency include:
- Explainable AI (XAI): Providing users with clear explanations of how AI generates insights.
- Confidence scores: Sharing reliability metrics for AI-driven conclusions.
- Documentation: Maintaining detailed records of data sources and processing methods.
Regulatory Considerations
Complying with regulations is non-negotiable for ethical AI deployment. Key areas of focus include:
- Data protection laws: Ensuring compliance with GDPR, CCPA, and similar frameworks.
- Cross-border data handling: Addressing challenges in international data transfers.
- Audit readiness: Preparing for regulatory audits with transparent records and processes.
Best Practices for Ethical AI Deployment
Organizations can adopt these best practices to ensure ethical AI use in BI:
- Ethics committees: Establishing oversight teams to review AI deployment strategies.
- Bias mitigation: Regularly auditing AI models for biases in data and analysis.
- Stakeholder education: Training teams on the ethical implications of AI use.
Conclusion
As AI continues to revolutionize business intelligence, ensuring ethical practices is critical for maintaining trust, compliance, and long-term success. By adopting responsible scraping practices, enhancing transparency, and adhering to regulatory standards, organizations can harness AI's potential while upholding ethical integrity.