Understanding AI Confidence Scores in Business Research
Learn how AI assigns confidence scores to data, the role of uncertainty, and best practices for using these scores in business decisions.
Posted by

Related reading
How to Generate High-Quality Local Leads for Your Agency
Discover effective strategies and tools to generate qualified local leads for your agency. Learn how to automate lead generation, enrich prospect data, and convert more clients without extensive manual research.
Building Scalable Business Intelligence Systems
Discover the architecture behind scalable BI systems, including parallel processing, API design, and best practices for large-scale data collection.
Markdown to Intelligence: Structuring Web Content with AI
Explore how AI converts raw web data into structured business intelligence using HTML cleaning, Markdown conversion, and NLP-driven insights.
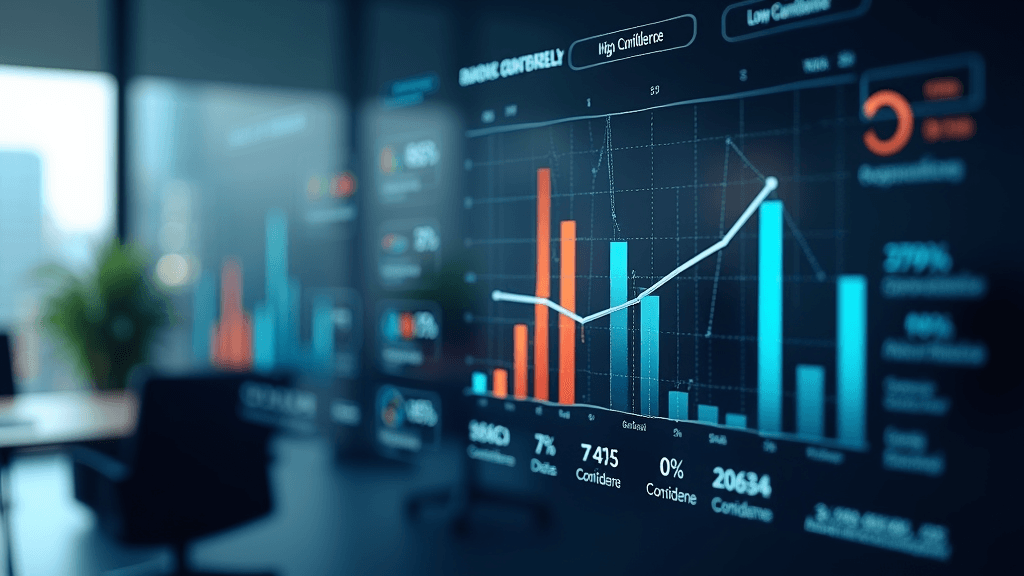
Introduction
Confidence scores in AI provide a quantifiable measure of certainty in the data extracted by machine learning models. These scores are integral to business research, offering insights into data reliability and supporting informed decision-making. In this article, we explore how confidence scores are generated, their role in AI-driven systems, and practical applications in business research.
What Are AI Confidence Scores?
AI confidence scores represent the system's level of certainty about a specific prediction or extracted data point. They are typically expressed as percentages, indicating how likely it is that the result is correct. These scores are derived from:
- Model outputs: The probability distributions generated by AI models during analysis.
- Data patterns: Patterns in training and validation datasets that influence certainty.
- Ensemble approaches: Combining predictions from multiple models to increase reliability.
Types of Uncertainty in AI Analysis
Confidence scores can vary based on two types of uncertainty:
- Aleatoric uncertainty: Arises from inherent data noise, such as missing or ambiguous information.
- Epistemic uncertainty: Stems from a lack of knowledge in the model itself, often due to limited training data for specific scenarios.
For instance, when analyzing company legal pages, aleatoric uncertainty may result from vague text, while epistemic uncertainty may arise if the AI has not encountered similar data before.
Confidence Scores in Practice
Modern business intelligence platforms, like AskMyBiz, use confidence scores to enhance data reliability. Key practices include:
- Dual-model validation: AskMyBiz uses Basic and Premium models, where the Premium model verifies and refines the results of the Basic model for sensitive tasks.
- Flagging low-confidence results: Results below a certain confidence threshold are flagged for manual review.
- Scoring sources: Assigning confidence scores to specific data sources based on their reliability and relevance.
Applications in Business Decisions
Confidence scores are invaluable in various business research scenarios:
- Due diligence: Confidence scores help identify high-risk areas in M&A, such as unverifiable compliance data.
- Market research: Scores ensure that trends and insights derived from competitor analysis are based on reliable data.
For example, during the screening of 5,000 companies, high-confidence scores enable faster identification of suitable acquisition targets.
Best Practices for Using Confidence Scores
To effectively use confidence scores in decision-making:
- Set thresholds: Define minimum acceptable scores for critical decisions.
- Combine human oversight: Pair AI insights with expert reviews for low-confidence results.
- Monitor and adjust: Regularly evaluate model performance to improve confidence calibration.
Conclusion
Confidence scores play a pivotal role in AI-driven business research by quantifying data reliability and identifying areas of uncertainty. By understanding how these scores are generated and using them effectively, businesses can make informed decisions, mitigate risks, and harness the full potential of AI insights.